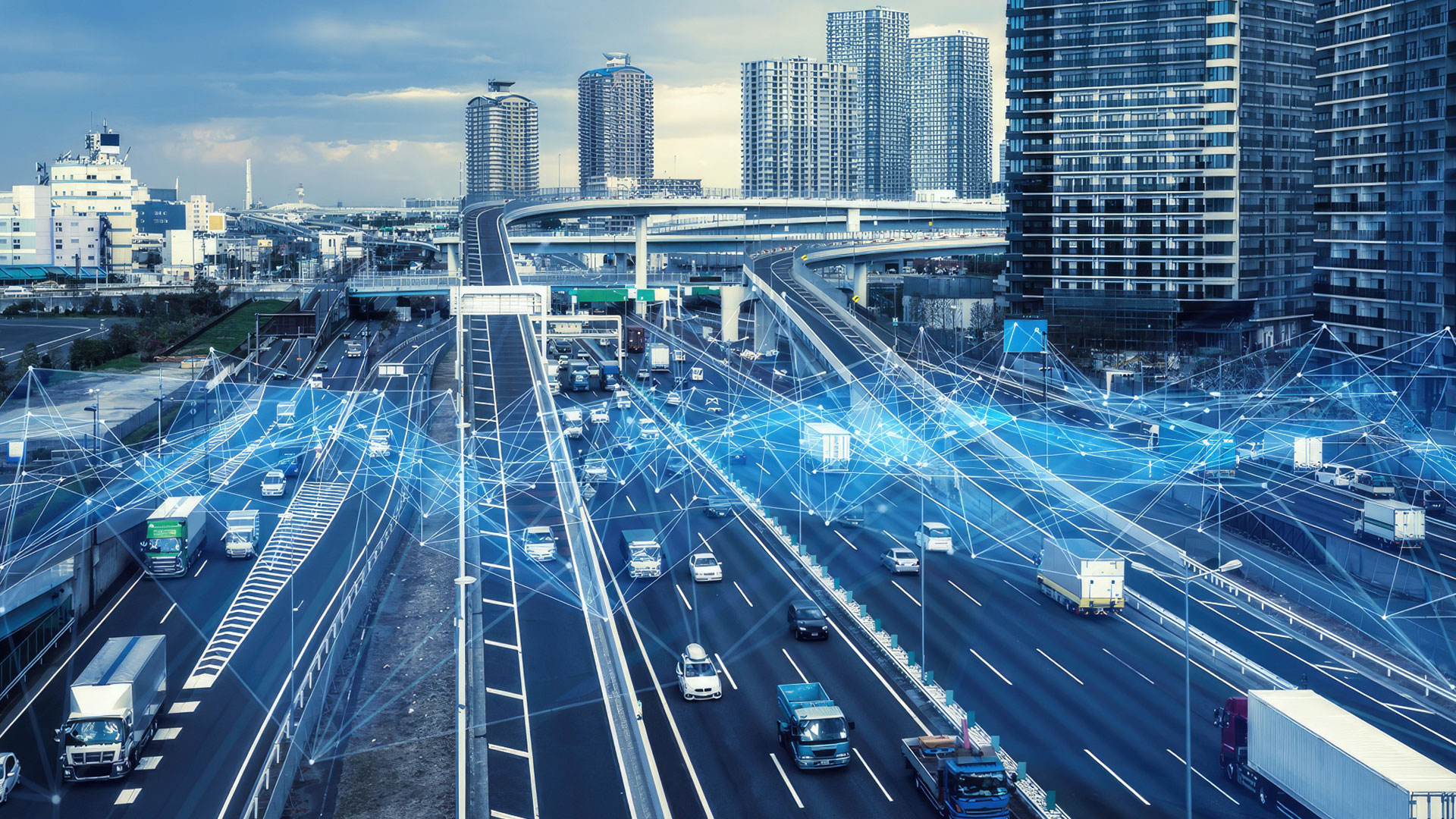
Picture this: Sami, a veteran trucker with miles of highway behind him, is stuck at a truck stop. He’s scrolling through load boards, making call after call, trying to find the right load. Hours slip by, and his truck is still empty. It’s a frustrating waste of time and fuel, a story too many truckers know too well.
Now, imagine a different scenario. With the power of AI, Sami gets the perfect load matched to his truck in minutes. No more endless searching, no more wasted miles. AI is transforming the logistics industry, revolutionizing load matching to make it faster, smarter, and incredibly efficient. The future of trucking is here, and it’s driven by artificial intelligence.
1. Load Matching Challenges
Scattered Information
The traditional process of load matching in the logistics industry is highly fragmented. Brokers and carriers rely on multiple load boards, phone calls, and emails to find and book loads. This scattered approach is time-consuming and inefficient, often resulting in significant delays and missed opportunities. Shippers post loads on various platforms, and carriers must constantly monitor multiple sources to find suitable matches, creating a chaotic and inefficient process.
Missed Opportunities
Manual load matching is riddled with limitations. Carriers frequently miss out on optimal loads due to the sheer volume of options and the lack of a centralized system. Similarly, shippers struggle to fill trucks efficiently, leading to suboptimal load matches that do not maximize truck capacity or route efficiency. This inefficiency not only affects individual carriers and shippers but also impacts the broader supply chain, causing delays and increased operational costs.
Empty Miles & Wasted Resources
One of the most significant issues in traditional load matching is the prevalence of empty miles—trucks driving without cargo. In the U.S., trucks drive empty for approximately 20-25% of their total mileage. This inefficiency leads to wasted fuel, increased emissions, and substantial revenue loss for carriers. According to industry data, this translates to billions of dollars in wasted fuel and operational costs annually, underscoring the urgent need for more efficient load-matching solutions.
Example: A report from the American Transportation Research Institute (ATRI) highlights that trucks in the U.S. average around 29% empty miles, representing a significant waste of resources and an opportunity for improvement through better load-matching technologies.
Load matching has always been a grind. It involves a lot of phone calls, faxes, and emails. Dispatchers spent hours coordinating between shippers and truckers, looking for the best fit for each load. It’s a tedious, manual process that relies heavily on human judgment and timing.
These methods are far from perfect. Delayed responses were common—missing a call could mean losing a load. Human error creeps in frequently, leading to mismatched loads where the truck size or delivery schedule doesn’t align with the shipper’s needs. The whole system is slow and prone to mistakes, creating frustration on all sides.
High-profile clients that demand punctual, reliable deliveries have a low tolerance for repeated delays and mismatched loads. In fact, they won’t even do business with logistics suppliers unless they can demonstrate that they have an efficient process to coordinate the delivery of their goods.
2. Introduction to AI in Logistics
Artificial Intelligence (AI) and machine learning are no longer just buzzwords—they’re game-changers in logistics. AI is increasingly performing tasks that usually require human intelligence, like decision-making and problem-solving. To be effective, AI needs human input to set the task and ensure that the bots are in fact producing the intended outcome.
Machine learning, on the other hand, involves algorithms that allow systems to learn from data and improve over time without being explicitly programmed.
AI is being used to tackle the industry’s unique challenges. It can analyze vast amounts of data in real time, optimizing routes, predicting maintenance needs, and most importantly, revolutionizing load matching. AI’s ability to process and integrate multiple data sources—from traffic patterns to weather forecasts—makes it indispensable for enhancing efficiency and reliability in logistics.
The Seattle-based startup, Convoy, was a leading example of AI in action until its recent shutdown. Founded in 2015, Convoy used AI to connect shippers with carriers, significantly improving load-matching efficiency and reducing empty miles by 45%.
Despite its promising technology and support from high-profile investors like Jeff Bezos and Bill Gates, Convoy couldn’t withstand the dual pressures of a freight recession and tightening capital markets.
While the company was forced to close its doors in October 2023, it highlighted the potential of AI-driven logistics. As the industry matures, AI and machine learning will yield better, more accurate results. The reality is that shippers want it, carriers, want it, and the market wants it.
3. How AI Transforms Load Matching
AI is revolutionizing load matching by integrating and analyzing data from multiple sources to make precise, real-time decisions, enhancing efficiency and reliability in the logistics industry.
Data Integration and Analysis
AI logistics systems combine data from various sources, including GPS, traffic updates, weather forecasts, and load boards. This creates a comprehensive picture of current conditions and predicts future scenarios. By analyzing this data, AI determines the best matches for loads and trucks, optimizing routes and schedules to minimize delays and maximize efficiency.
For instance, AI can predict traffic patterns and weather disruptions, adjusting routes in advance to avoid potential delays. This predictive capability ensures that loads are matched with the most suitable trucks, considering factors like truck size, load weight, and delivery deadlines.
Example: A real-world application of this is seen in the case of Loadsmart, a digital freight broker that uses AI to reduce empty miles. By leveraging AI, Loadsmart has cut down empty miles by over 20%, significantly improving operational efficiency and reducing costs.
Machine Learning Magic
AI algorithms learn from vast amounts of data, including historical load patterns, traffic conditions, and carrier preferences. These algorithms get smarter over time, continually improving their predictions and adapting to new data. This allows AI to anticipate demand, optimize routes, and enhance overall efficiency in logistics operations.
Smarter Matching, Faster Results
AI can match loads with trucks in real-time, taking into account a wide range of factors beyond just origin and destination. It considers weight limits, truck types, driver qualifications, and fuel efficiency to ensure optimal matches. For instance, an AI system can match a refrigerated load with a truck equipped with a cooling unit and a driver certified to handle perishable goods. This comprehensive approach ensures that the right truck gets the right load, reducing downtime and increasing operational efficiency.
Example: Consider the story of a driver using the AI-powered app from Uber Freight. The app matched the driver with an optimal load within minutes, considering his location, the truck’s specifications, and the delivery requirements. This type of instant match is rapidly becoming the norm and is set to increase earnings, reduce empty miles, and turbocharge customer satisfaction.
Dynamic Optimization
AI excels in dynamic optimization, adjusting routes and schedules in real time based on changing conditions like traffic jams, weather disruptions, or last-minute cancellations. This capability minimizes empty miles and ensures that deliveries are completed as efficiently as possible. Dynamic route optimization not only saves fuel and reduces emissions but also maximizes the utilization of each truck, boosting profitability for carriers..
Example: Dynamic route optimization uses real-time data to adjust delivery routes on the fly. If a truck encounters unexpected traffic, the AI system reroutes it to avoid delays, ensuring timely deliveries and reducing unnecessary fuel consumption. This flexibility enhances the reliability and efficiency of logistics operations, making the most of every mile driven.
4. Benefits for Carriers: More Money, Less Hassle
Increased Revenue
AI helps carriers find better-paying loads and reduce empty miles, significantly boosting profits. By utilizing machine learning algorithms, AI can identify the most profitable routes and load combinations, ensuring that trucks are always carrying optimal loads. This reduces the number of empty miles driven, which directly translates to higher revenue for carriers. According to industry sources, AI has enabled companies like Loadsmart to cut empty miles by over 20%, significantly enhancing operational efficiency and profitability.
Improved Efficiency
AI optimizes routes and schedules, saving carriers both time and fuel. By analyzing real-time data on traffic conditions, weather, and other factors, AI can determine the most efficient routes for deliveries. This dynamic route optimization not only ensures timely deliveries but also reduces fuel consumption and operational costs. Companies like Uber Freight have implemented AI to improve route planning, leading to significant time and cost savings.
Enhanced Visibility
AI platforms provide carriers with real-time updates on loads and market conditions, enhancing operational transparency. Mobile apps and AI-powered shipment tracking systems allow carriers to monitor their shipments in real time, adjust routes based on current conditions, and communicate effectively with shippers. This enhanced visibility helps carriers make informed decisions, improving reliability and customer satisfaction.
5. Benefits for Shippers: Efficiency Gains and Cost Savings
Faster Load Coverage
AI helps shippers find qualified carriers quickly, reducing wait times and ensuring on-time deliveries. By automating the matching process, AI can rapidly identify the best carrier for a specific load, streamlining operations and enhancing efficiency. This capability is particularly valuable in high-demand situations where quick turnaround is crucial.
Reduced Transportation Costs
AI optimizes routes and minimizes empty miles, leading to lower transportation costs for shippers. By using AI to analyze and predict the most efficient routes, shippers can significantly reduce fuel consumption and operational expenses. For example, Uber Freight’s AI solutions have helped shippers cut transportation costs by improving route efficiency and reducing empty miles.
Improved Communication and Transparency
AI platforms facilitate better communication between shippers and carriers, ensuring that all parties are informed and aligned throughout the shipping process. Features like automated documentation, real-time tracking, and communication tools enhance transparency and collaboration, leading to smoother operations and higher satisfaction rates. Shippers benefit from AI-driven insights that help them manage their supply chains more effectively and respond swiftly to any issues that arise..
These advancements in AI technology are transforming the logistics industry, making it more efficient, cost-effective, and reliable for both carriers and shippers.
6. Challenges and Considerations
Integration with Existing Systems
Technical Barriers: Integrating AI with legacy systems presents significant technical challenges. Many logistics companies operate on outdated infrastructure that isn’t compatible with modern AI solutions. This often necessitates extensive modifications and updates to existing systems to support AI integration.
Cost and Investment: The initial investment required for AI technology is substantial. Companies need to invest in new hardware, software, and employee training to effectively utilize AI systems. This financial burden can be daunting, particularly for smaller businesses. For example, UPS has invested heavily in AI, leveraging it to optimize routes, improve delivery times, and enhance customer satisfaction through systems like ORION and RFID technology for vaccine tracking during the pandemic.
Data Privacy and Security
Handling Sensitive Information: Data security is critical when implementing AI in logistics, as these systems handle vast amounts of sensitive information. Ensuring robust security measures to prevent data breaches is essential.
Compliance with Regulations: Companies must comply with data protection regulations such as the GDPR in Europe and the CCPA in California, which dictate how personal data should be handled and protected.
Conclusion
AI in load matching offers significant benefits, including increased efficiency, reduced costs, and enhanced operational reliability. However, it also presents challenges such as integration with legacy systems and ensuring data security.
Logistics companies should consider adopting AI to stay competitive and meet the growing demands of the market.
The potential of AI to revolutionize the logistics industry is immense, and those who invest in these technologies now will be well-positioned for future success
About Wahyd Logistics
Learn more about Wahyd Logistics technology-enabled logistics marketplace here. Allowing you to book, track, and manage your shipments with the touch of a button, our transparent, end-to-end logistics management solution caters to a number of industries worldwide.